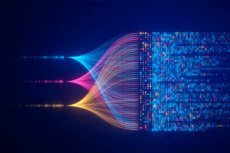
Machine learning (ML) methods can quickly and accurately diagnose mutations in gliomas - primary brain tumors.
This is confirmed by a recent study conducted by the Karl Landsteiner University of Medical Sciences (KL Krems). In this study, physiometabolic magnetic resonance imaging (MRI) data were analyzed using ML methods to identify mutations in a metabolic gene. Mutations in this gene have a significant impact on the course of the disease, and early diagnosis is important for treatment. The study also shows that there are currently inconsistent standards for obtaining physiometabolic MR images, which hinders routine clinical use of the method.
Gliomas are the most common primary brain tumors. Despite the still poor prognosis, personalized therapies can significantly improve treatment success. However, the use of such advanced therapies relies on individual tumor data, which is difficult to obtain for gliomas due to their location in the brain. Imaging techniques such as Magnetic resonance imaging (MRI) can provide such data, but their analysis is complex, labor-intensive, and time-consuming. The Central Institute for Diagnostic Medical Radiology at the University Hospital St. Pölten, the teaching and research base of KL Krems, has been developing machine and deep learning methods for many years to automate such analyzes and integrate them into routine clinical operations. Now another breakthrough has been achieved.
"Patients whose glioma cells carry a mutated form of the isocitrate dehydrogenase (IDH) gene actually have better clinical prospects than those with the wild type," explains Professor Andreas Stadlbauer, a medical physicist at the Central Institute. "This means that the sooner we know the mutation status, the better we can individualize treatment." Differences in the energy metabolism of mutated and wild-type tumors help with this. Thanks to previous work by Professor Stadlbauer's team, they can be easily measured using physiometabolic MRI, even without tissue samples. However, data analysis and evaluation is a very complex and time-consuming process that is difficult to integrate into clinical practice, especially because results are needed quickly due to the poor prognosis of patients.
In the current study, the team used ML methods to analyze and interpret this data to get results faster and be able to initiate appropriate treatment steps. But how accurate are the results? To evaluate this, the study first used data from 182 patients at the University Hospital St. Pölten, whose MRI data were collected using standardized protocols.
“When we saw the evaluation results of our ML algorithms,” explains Professor Stadlbauer, “we were very pleased. We achieved an accuracy of 91.7% and an accuracy of 87.5% in distinguishing tumors with the gene wild type from those with mutated form. We then compared these values with ML analyzes of classical clinical MRI data and were able to show that using physiometabolic MRI data as a basis produced significantly better results."
However, this superiority was only maintained when analyzing data collected in St. Pölten using a standardized protocol. This was not the case when the ML method was applied to external data, i.e., MRI data from other hospital databases. In this situation, the ML method trained on classical clinical MRI data was more successful.
The reason why analysis of physiometabolic MRI data using ML performed worse is that the technology is still young and in the experimental stage of development. Data collection methods still vary from hospital to hospital, leading to bias in ML analysis.
For the scientist, the problem is “only” the standardization that will inevitably arise with the increasing use of physiometabolic MRI in different hospitals. The method itself—rapid assessment of physiometabolic MRI data using ML methods—has shown excellent results. Therefore, this is an excellent approach to determine IDH mutation status in glioma patients preoperatively and to individualize treatment options.
The study results were published in the journal Karl Landsteiner University of Health Sciences (KL Krems).